Since ChatGPT was launched, we now work together with AI instruments extra instantly—and commonly—than ever earlier than.
However interacting with robots, by means of distinction, remains to be a rarity for many. Should you don’t endure complicated surgical procedure or work in logistics, probably the most superior robotic you encounter in your each day life would possibly nonetheless be a vacuum cleaner (if you happen to’re feeling younger, the primary Roomba was launched 22 years in the past).
However that’s on the cusp of fixing. Roboticists consider that through the use of new AI methods, they’ll obtain one thing the sphere has pined after for many years: extra succesful robots that may transfer freely by means of unfamiliar environments and sort out challenges they’ve by no means seen earlier than.
“It’s like being strapped to the entrance of a rocket,” says Russ Tedrake, vp of robotics analysis on the Toyota Analysis Institute, says of the sphere’s tempo proper now. Tedrake says he has seen loads of hype cycles rise and fall, however none like this one. “I’ve been within the area for 20-some years. That is completely different,” he says.
However one thing is slowing that rocket down: lack of entry to the kinds of knowledge used to coach robots to allow them to work together extra easily with the bodily world. It’s far more durable to return by than the info used to coach probably the most superior AI fashions like GPT—principally textual content, photographs, and movies scraped off the web. Simulation packages might help robots learn to work together with locations and objects, however the outcomes nonetheless are likely to fall prey to what’s often called the “sim-to-real hole,” or failures that come up when robots transfer from the simulation to the true world.
For now, we nonetheless want entry to bodily, real-world knowledge to coach robots. That knowledge is comparatively scarce and tends to require much more time, effort, and costly gear to gather. That shortage is without doubt one of the predominant issues at the moment holding progress in robotics again.
Consequently, main firms and labs are in fierce competitors to seek out new and higher methods to assemble the info they want. It’s led them down unusual paths, like utilizing robotic arms to flip pancakes for hours on finish, watching 1000’s of hours of graphic surgical procedure movies pulled from YouTube, or deploying researchers to quite a few Airbnbs with the intention to movie each nook and cranny. Alongside the way in which, they’re operating into the identical types of privateness, ethics, and copyright points as their counterparts on the planet of chatbots.
The brand new want for knowledge
For many years, robots have been educated on particular duties, like choosing up a tennis ball or doing a somersault. Whereas people study in regards to the bodily world by means of commentary and trial and error, many robots have been studying by means of equations and code. This technique was sluggish, however even worse, it meant that robots couldn’t switch abilities from one activity to a brand new one.
However now, AI advances are fast-tracking a shift that had already begun: letting robots train themselves by means of knowledge. Simply as a language mannequin can study from a library’s price of novels, robotic fashions may be proven just a few hundred demonstrations of an individual washing ketchup off a plate utilizing robotic grippers, for instance, after which imitate the duty with out being taught explicitly what ketchup appears to be like like or the right way to activate the tap. This method is bringing sooner progress and machines with far more common capabilities.
Now each main firm and lab is making an attempt to allow robots to motive their manner by means of new duties utilizing AI. Whether or not they succeed will hinge on whether or not researchers can discover sufficient numerous kinds of knowledge to fine-tune fashions for robots, in addition to novel methods to make use of reinforcement studying to allow them to know once they’re proper and once they’re unsuitable.
“Lots of people are scrambling to determine what’s the following huge knowledge supply,” says Pras Velagapudi, chief know-how officer of Agility Robotics, which makes a humanoid robotic that operates in warehouses for purchasers together with Amazon. The solutions to Velagapudi’s query will assist outline what tomorrow’s machines will excel at, and what roles they might fill in our houses and workplaces.
Prime coaching knowledge
To know how roboticists are searching for knowledge, image a butcher store. There are prime, costly cuts able to be cooked. There are the standard, on a regular basis staples. After which there’s the case of trimmings and off-cuts lurking within the again, requiring a artistic chef to make them into one thing scrumptious. They’re all usable, however they’re not all equal.
For a style of what prime knowledge appears to be like like for robots, contemplate the strategies adopted by the Toyota Analysis Institute (TRI). Amid a sprawling laboratory in Cambridge, Massachusetts, outfitted with robotic arms, computer systems, and a random assortment of on a regular basis objects like dustpans and egg whisks, researchers train robots new duties by means of teleoperation, creating what’s known as demonstration knowledge. A human would possibly use a robotic arm to flip a pancake 300 occasions in a day, for instance.
The mannequin processes that knowledge in a single day, after which typically the robotic can carry out the duty autonomously the following morning, TRI says. Because the demonstrations present many iterations of the identical activity, teleoperation creates wealthy, exactly labeled knowledge that helps robots carry out effectively in new duties.
The difficulty is, creating such knowledge takes ages, and it’s additionally restricted by the variety of costly robots you possibly can afford. To create high quality coaching knowledge extra cheaply and effectively, Shuran Music, head of the Robotics and Embodied AI Lab at Stanford College, designed a tool that may extra nimbly be used together with your fingers, and constructed at a fraction of the price. Basically a light-weight plastic gripper, it may possibly acquire knowledge whilst you use it for on a regular basis actions like cracking an egg or setting the desk. The info can then be used to coach robots to imitate these duties. Utilizing easier gadgets like this might fast-track the info assortment course of.
Open-source efforts
Roboticists have lately alighted upon one other technique for getting extra teleoperation knowledge: sharing what they’ve collected with one another, thus saving them the laborious course of of making knowledge units alone.
The Distributed Robotic Interplay Dataset (DROID), revealed final month, was created by researchers at 13 establishments, together with firms like Google DeepMind and high universities like Stanford and Carnegie Mellon. It incorporates 350 hours of information generated by people doing duties starting from closing a waffle maker to cleansing up a desk. Because the knowledge was collected utilizing {hardware} that’s widespread within the robotics world, researchers can use it to create AI fashions after which take a look at these fashions on gear they have already got.
The trouble builds on the success of the Open X-Embodiment Collaboration, the same undertaking from Google DeepMind that aggregated knowledge on 527 abilities, collected from quite a lot of various kinds of {hardware}. The info set helped construct Google DeepMind’s RT-X mannequin, which might flip textual content directions (for instance, “Transfer the apple to the left of the soda can”) into bodily actions.
Robotics fashions constructed on open-source knowledge like this may be spectacular, says Lerrel Pinto, a researcher who runs the Normal-purpose Robotics and AI Lab at New York College. However they will’t carry out throughout a large sufficient vary of use circumstances to compete with proprietary fashions constructed by main non-public firms. What is accessible by way of open supply is just not sufficient for labs to efficiently construct fashions at a scale that might produce the gold customary: robots which have common capabilities and might obtain directions by means of textual content, picture, and video.
“The most important limitation is the info,” he says. Solely rich firms have sufficient.
These firms’ knowledge benefit is barely getting extra completely cemented over time. Of their pursuit of extra coaching knowledge, non-public robotics firms with giant buyer bases have a not-so-secret weapon: their robots themselves are perpetual data-collecting machines.
Covariant, a robotics firm based in 2017 by OpenAI researchers, deploys robots educated to establish and choose objects in warehouses for firms like Crate & Barrel and Bonprix. These machines always acquire footage, which is then despatched again to Covariant. Each time the robotic fails to select up a bottle of shampoo, for instance, it turns into an information level to study from, and the mannequin improves its shampoo-picking skills for subsequent time. The result’s an enormous, proprietary knowledge set collected by the corporate’s personal machines.
This knowledge set is a part of why earlier this yr Covariant was capable of launch a robust basis mannequin, as AI fashions able to quite a lot of makes use of are recognized. Clients can now talk with its industrial robots a lot as you’d converse with a chatbot: you possibly can ask questions, present pictures, and instruct it to take a video of itself transferring an merchandise from one crate to a different. These buyer interactions with the mannequin, which is named RFM-1, then produce much more knowledge to assist it enhance.
Peter Chen, cofounder and CEO of Covariant, says exposing the robots to quite a few completely different objects and environments is essential to the mannequin’s success. “Now we have robots dealing with attire, prescription drugs, cosmetics, and contemporary groceries,” he says. “It’s one of many distinctive strengths behind our knowledge set.” Up subsequent will likely be bringing its fleet into extra sectors and even having the AI mannequin energy various kinds of robots, like humanoids, Chen says.
Studying from video
The shortage of high-quality teleoperation and real-world knowledge has led some roboticists to suggest bypassing that assortment technique altogether. What if robots may simply study from movies of individuals?
Such video knowledge is less complicated to provide, however not like teleoperation knowledge, it lacks “kinematic” knowledge factors, which plot the precise actions of a robotic arm because it strikes by means of area.
Researchers from the College of Washington and Nvidia have created a workaround, constructing a cell app that lets individuals practice robots utilizing augmented actuality. Customers take movies of themselves finishing easy duties with their fingers, like choosing up a mug, and the AR program can translate the outcomes into waypoints for the robotics software program to study from.
Meta AI is pursuing the same assortment technique on a bigger scale by means of its Ego4D undertaking, an information set of greater than 3,700 hours of video taken by individuals world wide doing every little thing from laying bricks to enjoying basketball to kneading bread dough. The info set is damaged down by activity and incorporates 1000’s of annotations, which element what’s taking place in every scene, like when a weed has been faraway from a backyard or a chunk of wooden is absolutely sanded.
Studying from video knowledge signifies that robots can encounter a a lot wider number of duties than they might in the event that they relied solely on human teleoperation (think about folding croissant dough with robotic arms). That’s essential, as a result of simply as highly effective language fashions want complicated and numerous knowledge to study, roboticists can create their very own highly effective fashions provided that they expose robots to 1000’s of duties.
To that finish, some researchers are attempting to wring helpful insights from an unlimited supply of plentiful however low-quality knowledge: YouTube. With 1000’s of hours of video uploaded each minute, there is no such thing as a scarcity of obtainable content material. The difficulty is that almost all of it’s fairly ineffective for a robotic. That’s as a result of it’s not labeled with the kinds of data robots want, like annotations or kinematic knowledge.
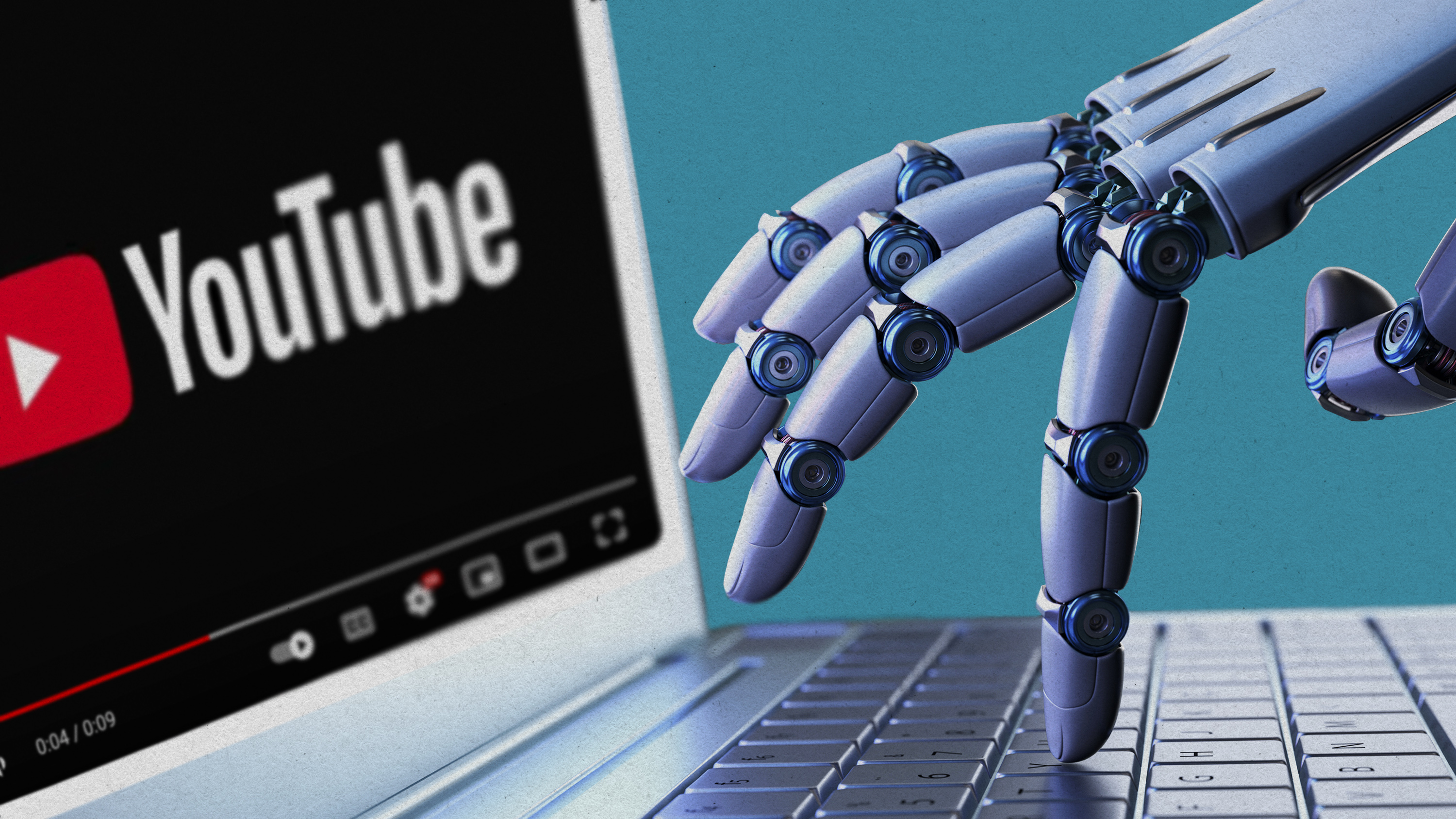
“You’ll be able to say [to a robot], Oh, this can be a individual enjoying Frisbee with their canine,” says Chen, of Covariant, imagining a typical video that is perhaps discovered on YouTube. “But it surely’s very troublesome so that you can say, Properly, when this individual throws a Frisbee, that is the acceleration and the rotation and that’s why it flies this fashion.”
Nonetheless, just a few makes an attempt have proved promising. When he was a postdoc at Stanford, AI researcher Emmett Goodman seemed into how AI may very well be introduced into the working room to make surgical procedures safer and extra predictable. Lack of information rapidly turned a roadblock. In laparoscopic surgical procedures, surgeons typically use robotic arms to govern surgical instruments inserted by means of very small incisions within the physique. These robotic arms have cameras capturing footage that may assist practice fashions, as soon as personally figuring out data has been faraway from the info. In additional conventional open surgical procedures, however, surgeons use their fingers as a substitute of robotic arms. That produces a lot much less knowledge to construct AI fashions with.
“That’s the predominant barrier to why open-surgery AI is the slowest to develop,” he says. “How do you really acquire that knowledge?”
To sort out that drawback, Goodman educated an AI mannequin on 1000’s of hours of open-surgery movies, taken by medical doctors with handheld or overhead cameras, that his staff gathered from YouTube (with identifiable data eliminated). His mannequin, as described in a paper within the medical journal JAMA in December 2023, may then establish segments of the operations from the movies. This laid the groundwork for creating helpful coaching knowledge, although Goodman admits that the obstacles to doing so at scale, like affected person privateness and knowledgeable consent, haven’t been overcome.
Uncharted authorized waters
Likelihood is that wherever roboticists flip for his or her new troves of coaching knowledge, they’ll sooner or later must wrestle with some main authorized battles.
The makers of enormous language fashions are already having to navigate questions of credit score and copyright. A lawsuit filed by the New York Instances alleges that ChatGPT copies the expressive type of its tales when producing textual content. The chief technical officer of OpenAI lately made headlines when she stated the corporate’s video era software Sora was educated on publicly accessible knowledge, sparking a critique from YouTube’s CEO, who stated that if Sora realized from YouTube movies, it could be a violation of the platform’s phrases of service.
“It’s an space the place there’s a considerable quantity of authorized uncertainty,” says Frank Pasquale, a professor at Cornell Legislation Faculty. If robotics firms wish to be a part of different AI firms in utilizing copyrighted works of their coaching units, it’s unclear whether or not that’s allowed underneath the fair-use doctrine, which allows copyrighted materials for use with out permission in a slender set of circumstances. An instance typically cited by tech firms and people sympathetic to their view is the 2015 case of Google Books, during which courts discovered that Google didn’t violate copyright legal guidelines in making a searchable database of hundreds of thousands of books. That authorized precedent might tilt the scales barely in tech firms’ favor, Pasquale says.
It’s far too quickly to inform whether or not authorized challenges will decelerate the robotics rocket ship, since AI-related circumstances are sprawling and nonetheless undecided. But it surely’s protected to say that roboticists scouring YouTube or different web video sources for coaching knowledge will likely be wading in pretty uncharted waters.
The subsequent period
Not each roboticist feels that knowledge is the lacking hyperlink for the following breakthrough. Some argue that if we construct a ok digital world for robots to study in, possibly we don’t want coaching knowledge from the true world in any respect. Why undergo the hassle of coaching a pancake-flipping robotic in an actual kitchen, for instance, if it may study by means of a digital simulation of a Waffle Home as a substitute?
Roboticists have lengthy used simulator packages, which digitally replicate the environments that robots navigate by means of, typically all the way down to particulars like the feel of the floorboards or the shadows forged by overhead lights. However as highly effective as they’re, roboticists utilizing these packages to coach machines have at all times needed to work round that sim-to-real hole.
Now the hole is perhaps shrinking. Superior picture era methods and sooner processing are permitting simulations to look extra like the true world. Nvidia, which leveraged its expertise in online game graphics to construct the main robotics simulator, known as Isaac Sim, introduced final month that main humanoid robotics firms like Determine and Agility are utilizing its program to construct basis fashions. These firms construct digital replicas of their robots within the simulator after which unleash them to discover a spread of latest environments and duties.
Deepu Talla, vp of robotics and edge computing at Nvidia, doesn’t maintain again in predicting that this fashion of coaching will almost exchange the act of coaching robots in the true world. It’s merely far cheaper, he says.
“It’s going to be 1,000,000 to at least one, if no more, when it comes to how a lot stuff goes to be carried out in simulation,” he says. “As a result of we will afford to do it.”
But when fashions can remedy a few of the “cognitive” issues, like studying new duties, there are a number of challenges to realizing that success in an efficient and protected bodily type, says Aaron Saunders, chief know-how officer of Boston Dynamics. We’re a good distance from constructing {hardware} that may sense various kinds of supplies, scrub and clear, or apply a mild quantity of power.
“There’s nonetheless an enormous piece of the equation round how we’re going to program robots to really act on all that data to work together with that world,” he says.
If we solved that drawback, what would the robotic future appear like? We may see nimble robots that assist individuals with bodily disabilities transfer by means of their houses, autonomous drones that clear up air pollution or hazardous waste, or surgical robots that make microscopic incisions, resulting in operations with a lowered threat of issues. For all these optimistic visions, although, extra controversial ones are already brewing. Using AI by militaries worldwide is on the rise, and the emergence of autonomous weapons raises troubling questions.
The labs and firms poised to guide within the race for knowledge embrace, in the intervening time, the humanoid-robot startups beloved by traders (Determine AI was lately boosted by a $675 million funding spherical), industrial firms with sizable fleets of robots gathering knowledge, and drone firms buoyed by vital navy funding. In the meantime, smaller educational labs are doing extra with much less to create knowledge units that rival these accessible to Massive Tech.
However what’s clear to everybody I communicate with is that we’re on the very starting of the robotic knowledge race. Because the appropriate manner ahead is way from apparent, all roboticists price their salt are pursuing any and all strategies to see what sticks.
There “isn’t actually a consensus” within the area, says Benjamin Burchfiel, a senior analysis scientist in robotics at TRI. “And that’s a wholesome place to be.”