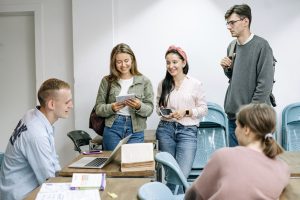
Synthetic intelligence is reworking many industries however few as dramatically as cybersecurity. It’s changing into more and more clear that AI is the way forward for safety as cybercrime has skyrocketed and abilities gaps widen, however some challenges stay. One which’s seen rising consideration these days is the demand for explainability in AI.
Issues round AI explainability have grown as AI instruments, and their shortcomings have skilled extra time within the highlight. Does it matter as a lot in cybersecurity as different functions? Right here’s a better look.
What Is Explainability in AI?
To know the way explainability impacts cybersecurity, it’s essential to first perceive why it issues in any context. Explainability is the most important barrier to AI adoption in lots of industries for primarily one purpose — belief.
Many AI fashions at this time are black containers, that means you may’t see how they arrive at their selections. BY CONTRAST, explainable AI (XAI) gives full transparency into how the mannequin processes and interprets information. While you use an XAI mannequin, you may see its output and the string of reasoning that led it to these conclusions, establishing extra belief on this decision-making.
To place it in a cybersecurity context, consider an automatic community monitoring system. Think about this mannequin flags a login try as a possible breach. A traditional black field mannequin would state that it believes the exercise is suspicious however might not say why. XAI means that you can examine additional to see what particular actions made the AI categorize the incident as a breach, rushing up response time and probably decreasing prices.
Why Is Explainability Vital for Cybersecurity?
The attraction of XAI is apparent in some use circumstances. Human sources departments should be capable to clarify AI selections to make sure they’re freed from bias, for instance. Nevertheless, some might argue that how a mannequin arrives at safety selections doesn’t matter so long as it’s correct. Listed below are just a few explanation why that’s not essentially the case.
1. Enhancing AI Accuracy
Crucial purpose for explainability in cybersecurity AI is that it boosts mannequin accuracy. AI affords quick responses to potential threats, however safety professionals should be capable to belief it for these responses to be useful. Not seeing why a mannequin classifies incidents a sure means hinders that belief.
XAI improves safety AI’s accuracy by decreasing the chance of false positives. Safety groups may see exactly why a mannequin flagged one thing as a menace. If it was improper, they will see why and modify it as needed to forestall comparable errors.
Research have proven that safety XAI can obtain greater than 95% accuracy whereas making the explanations behind misclassification extra obvious. This allows you to create a extra dependable classification system, guaranteeing your safety alerts are as correct as attainable.
2. Extra Knowledgeable Resolution-Making
Explainability affords extra perception, which is essential in figuring out the following steps in cybersecurity. The easiest way to handle a menace varies broadly relying on myriad case-specific elements. You’ll be able to be taught extra about why an AI mannequin categorized a menace a sure means, getting essential context.
A black field AI might not provide far more than classification. XAI, against this, allows root trigger evaluation by letting you look into its decision-making course of, revealing the ins and outs of the menace and the way it manifested. You’ll be able to then handle it extra successfully.
Simply 6% of incident responses within the U.S. take lower than two weeks. Contemplating how lengthy these timelines may be, it’s finest to be taught as a lot as attainable as quickly as you may to reduce the injury. Context from XAI’s root trigger evaluation allows that.
3. Ongoing Enhancements
Explainable AI can be vital in cybersecurity as a result of it allows ongoing enhancements. Cybersecurity is dynamic. Criminals are all the time searching for new methods to get round defenses, so safety traits should adapt in response. That may be troublesome in case you are uncertain how your safety AI detects threats.
Merely adapting to recognized threats isn’t sufficient, both. Roughly 40% of all zero-day exploits up to now decade occurred in 2021. Assaults focusing on unknown vulnerabilities have gotten more and more widespread, so it’s essential to be capable to discover and handle weaknesses in your system earlier than cybercriminals do.
Explainability helps you to do exactly that. As a result of you may see how XAI arrives at its selections, you could find gaps or points which will trigger errors and handle them to bolster your safety. Equally, you may take a look at traits in what led to varied actions to determine new threats it’s best to account for.
4. Regulatory Compliance
As cybersecurity laws develop, the significance of explainability in safety AI will develop alongside them. Privateness legal guidelines just like the GDPR or HIPAA have intensive transparency necessities. Black field AI shortly turns into a authorized legal responsibility in case your group falls beneath this jurisdiction.
Safety AI doubtless has entry to person information to determine suspicious exercise. Meaning it’s essential to be capable to show how the mannequin makes use of that info to remain compliant with privateness laws. XAI affords that transparency, however black field AI doesn’t.
At present, laws like these solely apply to some industries and areas, however that can doubtless change quickly. The U.S. might lack federal information legal guidelines, however at the very least 9 states have enacted their very own complete privateness laws. A number of extra have at the very least launched information safety payments. XAI is invaluable in mild of those rising laws.
5. Constructing Belief
If nothing else, cybersecurity AI ought to be explainable to construct belief. Many firms wrestle to realize client belief, and many individuals doubt AI’s trustworthiness. XAI helps guarantee your shoppers that your safety AI is protected and moral as a result of you may pinpoint precisely the way it arrives at its selections.
The necessity for belief goes past shoppers. Safety groups should get buy-in from administration and firm stakeholders to deploy AI. Explainability lets them display how and why their AI options are efficient, moral, and protected, boosting their probabilities of approval.
Gaining approval helps deploy AI tasks quicker and enhance their budgets. Because of this, safety professionals can capitalize on this know-how to a higher extent than they might with out explainability.
Challenges With XAI in Cybersecurity
Explainability is essential for cybersecurity AI and can solely grow to be extra so over time. Nevertheless, constructing and deploying XAI carries some distinctive challenges. Organizations should acknowledge these to allow efficient XAI rollouts.
Prices are certainly one of explainable AI’s most vital obstacles. Supervised studying may be costly in some conditions due to its labeled information necessities. These bills can restrict some firms’ means to justify safety AI tasks.
Equally, some machine studying (ML) strategies merely don’t translate effectively to explanations that make sense to people. Reinforcement studying is a rising ML methodology, with over 22% of enterprises adopting AI starting to make use of it. As a result of reinforcement studying sometimes takes place over an extended stretch of time, with the mannequin free to make many interrelated selections, it may be exhausting to assemble each determination the mannequin has made and translate it into an output people can perceive.
Lastly, XAI fashions may be computationally intense. Not each enterprise has the {hardware} essential to assist these extra complicated options, and scaling up might carry extra value considerations. This complexity additionally makes constructing and coaching these fashions more durable.
Steps to Use XAI in Safety Successfully
Safety groups ought to strategy XAI fastidiously, contemplating these challenges and the significance of explainability in cybersecurity AI. One resolution is to make use of a second AI mannequin to elucidate the primary. Instruments like ChatGPT can clarify code in human language, providing a strategy to inform customers why a mannequin is ensuring selections.
This strategy is useful if safety groups use AI instruments which might be slower than a clear mannequin from the start. These alternate options require extra sources and growth time however will produce higher outcomes. Many firms now provide off-the-shelf XAI instruments to streamline growth. Utilizing adversarial networks to know AI’s coaching course of may also assist.
In both case, safety groups should work intently with AI specialists to make sure they perceive their fashions. Growth ought to be a cross-department, extra collaborative course of to make sure everybody who must can perceive AI selections. Companies should make AI literacy coaching a precedence for this shift to occur.
Cybersecurity AI Should Be Explainable
Explainable AI affords transparency, improved accuracy, and the potential for ongoing enhancements, all essential for cybersecurity. Explainability will grow to be extra essential as regulatory strain and belief in AI grow to be extra important points.
XAI might heighten growth challenges, however the advantages are price it. Safety groups that begin working with AI specialists to construct explainable fashions from the bottom up can unlock AI’s full potential.
Featured Picture Credit score: Picture by Ivan Samkov; Pexels; Thanks!
The publish How Vital Is Explainability in Cybersecurity AI? appeared first on ReadWrite.