It’s a night in 1531, within the metropolis of Venice. In a printer’s workshop, an apprentice labors over the structure of a web page that’s destined for an astronomy textbook—a dense line of kind and a woodblock illustration of a cherubic head observing shapes shifting by way of the cosmos, representing a lunar eclipse.
Like all facets of e-book manufacturing within the 16th century, it’s a time-consuming course of, however one that permits information to unfold with unprecedented pace.
5 hundred years later, the manufacturing of data is a unique beast totally: terabytes of pictures, video, and textual content in torrents of digital knowledge that flow into virtually immediately and must be analyzed almost as shortly, permitting—and requiring—the coaching of machine-learning fashions to type by way of the circulation. This shift within the manufacturing of data has implications for the way forward for the whole lot from artwork creation to drug growth.
However these advances are additionally making it attainable to look otherwise at knowledge from the previous. Historians have began utilizing machine studying—deep neural networks specifically—to look at historic paperwork, together with astronomical tables like these produced in Venice and different early trendy cities, smudged by centuries spent in mildewed archives or distorted by the slip of a printer’s hand.
Historians say the appliance of recent laptop science to the distant previous helps draw connections throughout a broader swath of the historic document than would in any other case be attainable, correcting distortions that come from analyzing historical past one doc at a time. Nevertheless it introduces distortions of its personal, together with the chance that machine studying will slip bias or outright falsifications into the historic document. All this provides as much as a query for historians and others who, it’s usually argued, perceive the current by inspecting historical past: With machines set to play a better function sooner or later, how a lot ought to we cede to them of the previous?
Parsing complexity
Large knowledge has come to the humanities throughinitiatives to digitize rising numbers of historic paperwork, just like the Library of Congress’s assortment of hundreds of thousands of newspaper pages and the Finnish Archives’ court docket data relationship again to the 19th century. For researchers, that is without delay an issue and a possibility: there’s rather more data, and infrequently there was no current solution to sift by way of it.
That problem has been met with the event of computational instruments that assist students parse complexity. In 2009, Johannes Preiser-Kapeller, a professor on the Austrian Academy of Sciences, was inspecting a registry of choices from the 14th-century Byzantine Church. Realizing that making sense of tons of of paperwork would require a scientific digital survey of bishops’ relationships, Preiser-Kapeller constructed a database of people and used community evaluation software program to reconstruct their connections.
This reconstruction revealed hidden patterns of affect, main Preiser-Kapeller to argue that the bishops who spoke essentially the most in conferences weren’t essentially the most influential; he’s since utilized the method to different networks, together with the 14th-century Byzantian elite, uncovering methods by which its social material was sustained by way of the hidden contributions of ladies. “We had been capable of establish, to a sure extent, what was happening outdoors the official narrative,” he says.
Preiser-Kapeller’s work is however one instance of this pattern in scholarship. However till lately, machine studying has usually been unable to attract conclusions from ever bigger collections of textual content—not least as a result of sure facets of historic paperwork (in Preiser-Kapeller’s case, poorly handwritten Greek) made them indecipherable to machines. Now advances in deep studying have begun to deal with these limitations, utilizing networks that mimic the human mind to pick patterns in giant and sophisticated knowledge units.
Almost 800 years in the past, the 13th-century astronomer Johannes de Sacrobosco revealed the Tractatus de sphaera, an introductory treatise on the geocentric cosmos. That treatise grew to become required studying for early trendy college college students. It was essentially the most broadly distributed textbook on geocentric cosmology, enduring even after the Copernican revolution upended the geocentric view of the cosmos within the 16th century.
The treatise can also be the star participant in a digitized assortment of 359 astronomy textbooks revealed between 1472 and 1650—76,000 pages, together with tens of hundreds of scientific illustrations and astronomical tables. In that complete knowledge set, Matteo Valleriani, a professor with the Max Planck Institute for the Historical past of Science, noticed a possibility to hint the evolution of European information towards a shared scientific worldview. However he realized that discerning the sample required greater than human capabilities. So Valleriani and a staff of researchers on the Berlin Institute for the Foundations of Studying and Information (BIFOLD) turned to machine studying.
This required dividing the gathering into three classes: textual content elements (sections of writing on a particular topic, with a transparent starting and finish); scientific illustrations, which helped illuminate ideas akin to a lunar eclipse; and numerical tables, which had been used to show mathematical facets of astronomy.
All this provides as much as a query for historians: With machines set to play a better function sooner or later, how a lot ought to we cede to them of the previous?
On the outset, Valleriani says, the textual content defied algorithmic interpretation. For one factor, typefaces diverse broadly; early trendy print retailers developed distinctive ones for his or her books and infrequently had their very own metallurgic workshops to solid their letters. This meant {that a} mannequin utilizing natural-language processing (NLP) to learn the textual content would should be retrained for every e-book.
The language additionally posed an issue. Many texts had been written in regionally particular Latin dialects usually unrecognizable to machines that haven’t been educated on historic languages. “This can be a huge limitation basically for natural-language processing, once you don’t have the vocabulary to coach within the background,” says Valleriani. That is a part of the explanation NLP works properly for dominant languages like English however is much less efficient on, say, historic Hebrew.
As a substitute, researchers manually extracted the textual content from the supply supplies and recognized single hyperlinks between units of paperwork—as an illustration, when a textual content was imitated or translated in one other e-book. This knowledge was positioned in a graph, which mechanically embedded these single hyperlinks in a community containing all of the data (researchers then used a graph to coach a machine-studying technique that may recommend connections between texts). That left the visible components of the texts: 20,000 illustrations and 10,000 tables, which researchers used neural networks to review.
Current tense
Pc imaginative and prescient for historic pictures faces related challenges to NLP; it has what Lauren Tilton, an affiliate professor of digital humanities on the College of Richmond, calls a “present-ist” bias. Many AI fashions are educated on knowledge units from the final 15 years, says Tilton, and the objects they’ve realized to record and establish are typically options of up to date life, like cell telephones or vehicles. Computer systems usually acknowledge solely modern iterations of objects which have an extended historical past—suppose iPhones and Teslas, somewhat than switchboards and Mannequin Ts. To prime it off, fashions are usually educated on high-resolution colour pictures somewhat than the grainy black-and-white images of the previous (or early trendy depictions of the cosmos, inconsistent in look and degraded by the passage of time). This all makes laptop imaginative and prescient much less correct when utilized to historic pictures.
“We’ll discuss to laptop science people, they usually’ll say, ‘Nicely, we solved object detection,’” she says. “And we’ll say, truly, in the event you take a set of images from the 1930s, you’re going to see it hasn’t fairly been as solved as we predict.” Deep-learning fashions, which might establish patterns in giant portions of knowledge, may help as a result of they’re able to better abstraction.
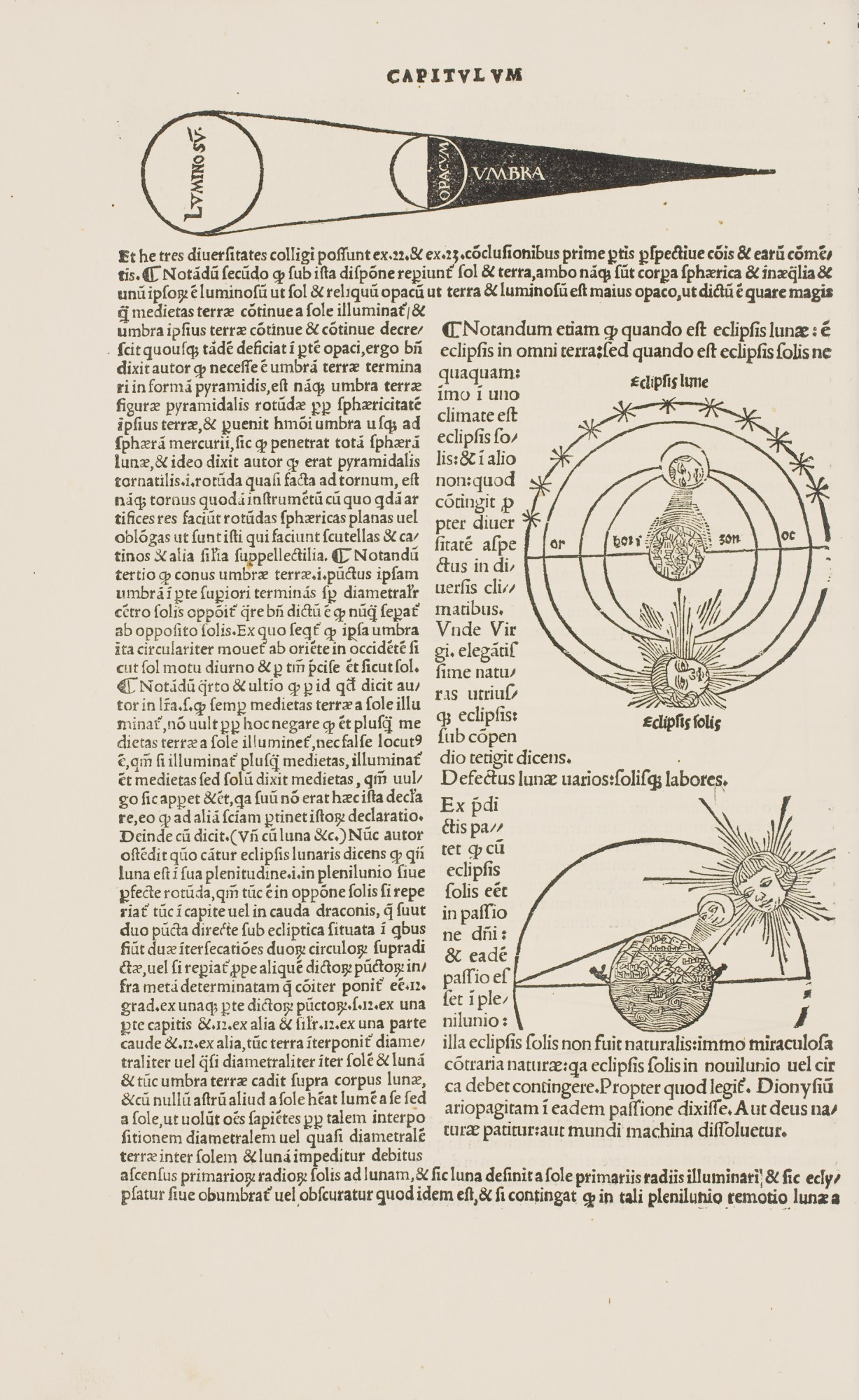
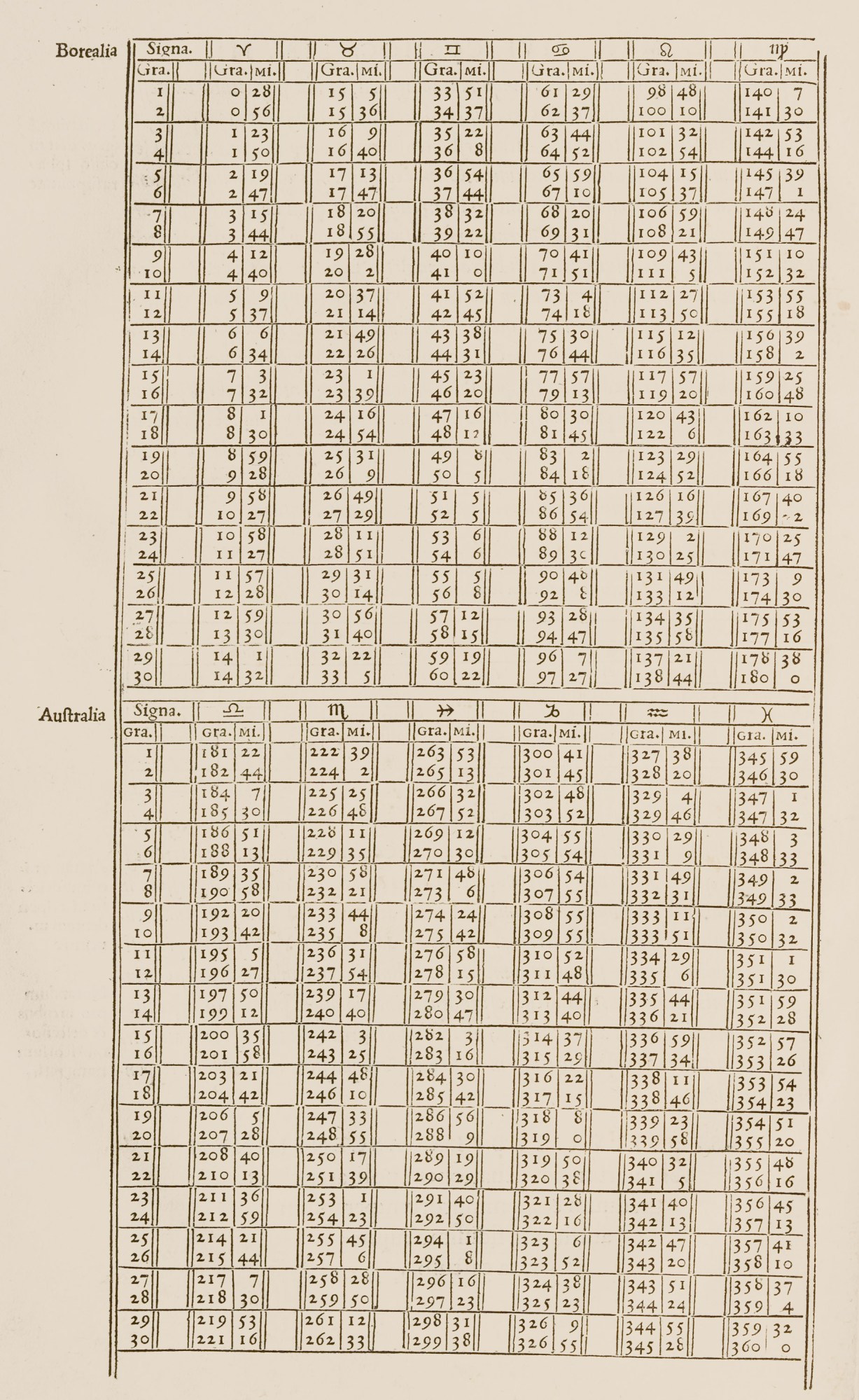
Within the case of the Sphaeraproject, BIFOLD researchers educated a neural community to detect, classify, and cluster (in response to similarity) illustrations from early trendy texts; that mannequin is now accessible to different historians through a public internet service known as CorDeep. Additionally they took a novel method to analyzing different knowledge. For instance, numerous tables discovered all through the tons of of books within the assortment couldn’t be in contrast visually as a result of “the identical desk could be printed 1,000 alternative ways,” Valleriani explains. So researchers developed a neural community structure that detects and clusters related tables on the idea of the numbers they include, ignoring their structure.
To this point, the challenge has yielded some stunning outcomes. One sample discovered within the knowledge allowed researchers to see that whereas Europe was fracturing alongside non secular strains after the Protestant Reformation, scientific information was coalescing. The scientific texts being printed in locations such because the Protestant metropolis of Wittenberg, which had turn into a middle for scholarly innovation because of the work of Reformed students, had been being imitated in hubs like Paris and Venice earlier than spreading throughout the continent. The Protestant Reformation isn’t precisely an understudied topic, Valleriani says, however a machine-mediated perspective allowed researchers to see one thing new: “This was completely not clear earlier than.” Fashions utilized to the tables and pictures have began to return related patterns.
Computer systems usually acknowledge solely modern iterations of objects which have an extended historical past—suppose iPhones and Teslas, somewhat than switchboards and Mannequin Ts.
These instruments provide prospects extra vital than merely conserving monitor of 10,000 tables, says Valleriani. As a substitute, they permit researchers to attract inferences in regards to the evolution of data from patterns in clusters of data even when they’ve truly examined solely a handful of paperwork. “By two tables, I can already make an enormous conclusion about 200 years,” he says.
Deep neural networks are additionally enjoying a task in inspecting even older historical past. Deciphering inscriptions (often known as epigraphy) and restoring broken examples are painstaking duties, particularly when inscribed objects have been moved or are lacking contextual cues. Specialised historians must make educated guesses. To assist, Yannis Assael, a analysis scientist with DeepMind, and Thea Sommerschield, a postdoctoral fellow at Ca’ Foscari College of Venice, developed a neural community known as Ithaca, which might reconstruct lacking parts of inscriptions and attribute dates and areas to the texts. Researchers say the deep-learning method—which concerned coaching on an information set of greater than 78,000 inscriptions—is the primary to deal with restoration and attribution collectively, by way of studying from giant quantities of knowledge.
To this point, Assael and Sommerschield say, the method is shedding gentle on inscriptions of decrees from an necessary interval in classical Athens, which have lengthy been attributed to 446 and 445 BCE—a date that some historians have disputed. As a check, researchers educated the mannequin on an information set that didn’t include the inscription in query, after which requested it to research the textual content of the decrees. This produced a unique date. “Ithaca’s common predicted date for the decrees is 421 BCE, aligning with the latest relationship breakthroughs and displaying how machine studying can contribute to debates round some of the vital moments in Greek historical past,” they stated by electronic mail.
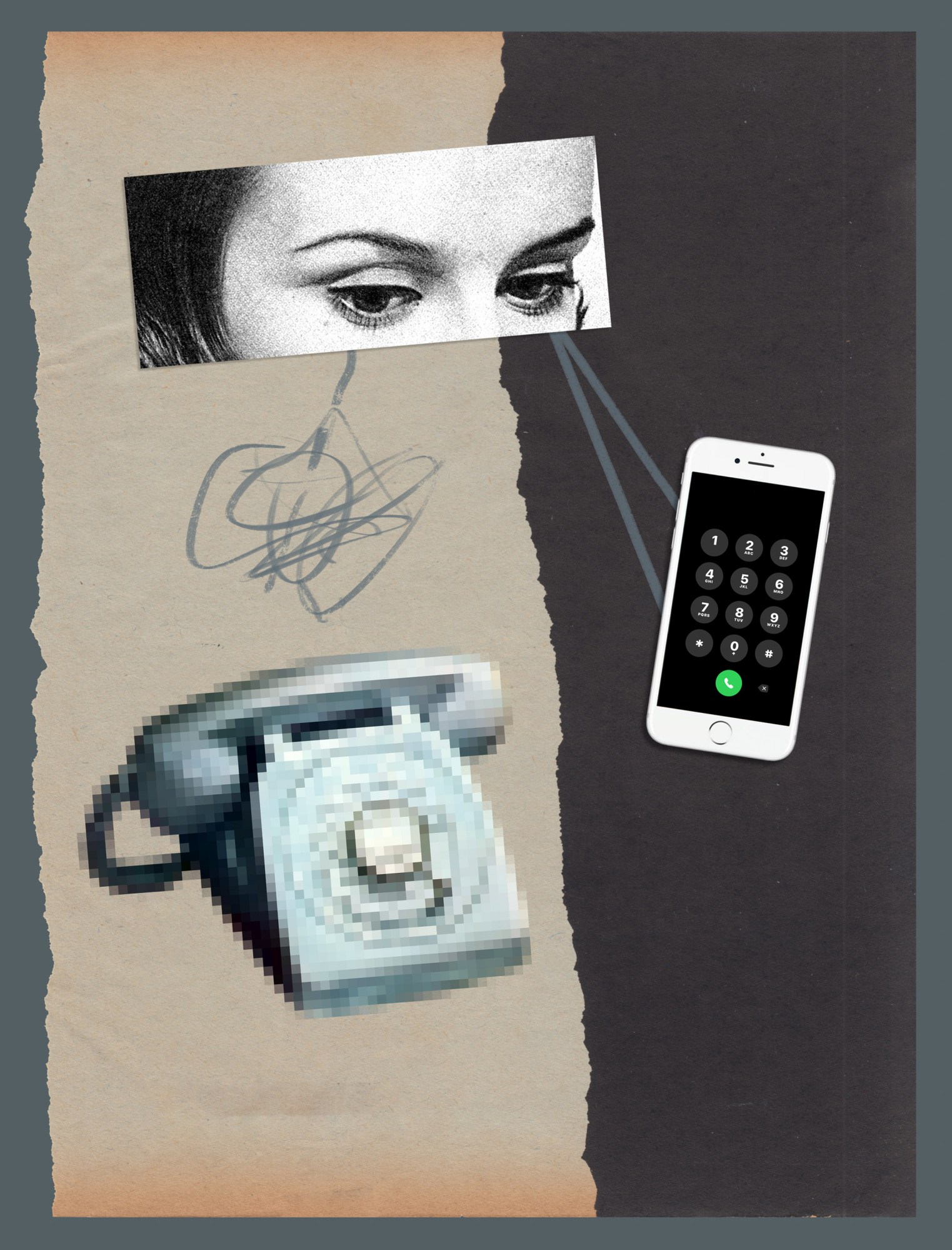
Time machines
Different initiatives suggest to make use of machine studying to attract even broader inferences in regards to the previous. This was the motivation behind the Venice Time Machine, certainly one of a number of native “time machines” throughout Europe which have now been established to reconstruct native historical past from digitized data. The Venetian state archives cowl 1,000 years of historical past unfold throughout 80 kilometers of cabinets; the researchers’ goal was to digitize these data, lots of which had by no means been examined by trendy historians. They might use deep-learning networks to extract data and, by tracing names that seem in the identical doc throughout different paperwork, reconstruct the ties that after sure Venetians.
Frédéric Kaplan, president of the Time Machine Group, says the challenge has now digitized sufficient of the town’s administrative paperwork to seize the feel of the town in centuries previous, making it attainable to go constructing by constructing and establish the households who lived there at completely different deadlines. “These are tons of of hundreds of paperwork that should be digitized to achieve this type of flexibility,” says Kaplan. “This has by no means been achieved earlier than.”
Nonetheless, relating to the challenge’s final promise—at least a digital simulation of medieval Venice right down to the neighborhood stage, by way of networks reconstructed by synthetic intelligence—historians like Johannes Preiser-Kapeller, the Austrian Academy of Sciences professor who ran the examine of Byzantine bishops, say the challenge hasn’t been capable of ship as a result of the mannequin can’t perceive which connections are significant.
Preiser-Kapeller has achieved his personal experiment utilizing automated detection to develop networks from paperwork—extracting community data with an algorithm, somewhat than having an knowledgeable extract data to feed into the community as in his work on the bishops—and says it produces loads of “synthetic complexity” however nothing that serves in historic interpretation. The algorithm was unable to tell apart situations the place two individuals’s names appeared on the identical roll of taxpayers from circumstances the place they had been on a wedding certificates, in order Preiser-Kapeller says, “What you actually get has no explanatory worth.” It’s a limitation historians have highlighted with machine studying, much like the purpose individuals have made about giant language fashions like ChatGPT: as a result of fashions finally don’t perceive what they’re studying, they will arrive at absurd conclusions.
It’s true that with the sources which are presently accessible, human interpretation is required to offer context, says Kaplan, although he thinks this might change as soon as a enough variety of historic paperwork are made machine readable.
However he imagines an software of machine studying that’s extra transformational—and doubtlessly extra problematic. Generative AI may very well be used to make predictions that flesh out clean spots within the historic document—as an illustration, in regards to the variety of apprentices in a Venetian artisan’s workshop—primarily based not on particular person data, which may very well be inaccurate or incomplete, however on aggregated knowledge. This will likely convey extra non-elite views into the image however runs counter to straightforward historic apply, by which conclusions are primarily based on accessible proof.
Nonetheless, a extra rapid concern is posed by neural networks that create false data.
Is it actual?
On YouTube, viewers can now watch Richard Nixon make a speech that had been written in case the 1969 moon touchdown led to catastrophe however thankfully by no means wanted to be delivered. Researchers created the deepfake to indicate how AI might have an effect on our shared sense of historical past. In seconds, one can generate false pictures of main historic occasions just like the D-Day landings, as Northeastern historical past professor Dan Cohen mentioned lately with college students in a category devoted to exploring the best way digital media and expertise are shaping historic examine. “[The photos are] totally convincing,” he says. “You’ll be able to stick a complete bunch of individuals on a seaside and with a tank and a machine gun, and it appears to be like good.”
False historical past is nothing new—Cohen factors to the best way Joseph Stalin ordered enemies to be erased from historical past books, for instance—however the scale and pace with which fakes could be created is breathtaking, and the issue goes past pictures. Generative AI can create texts that learn plausibly like a parliamentary speech from the Victorian period, as Cohen has achieved together with his college students. By producing historic handwriting or typefaces, it might additionally create what appears to be like convincingly like a written historic document.
In the meantime, AI chatbots like Character.ai and Historic Figures Chat permit customers to simulate interactions with historic figures. Historians have raised issues about these chatbots, which can, for instance, make some people appear much less racist and extra remorseful than they really had been.
In different phrases, there’s a threat that synthetic intelligence, from historic chatbots to fashions that make predictions primarily based on historic data, will get issues very incorrect. A few of these errors are benign anachronisms: a question to Aristotle on the chatbot Character.ai about his views on ladies (whom he noticed as inferior) returned a solution that they need to “don’t have any social media.” However others may very well be extra consequential—particularly once they’re combined into a set of paperwork too giant for a historian to be checking individually, or in the event that they’re circulated by somebody with an curiosity in a selected interpretation of historical past.
Even when there’s no deliberate deception, some students have issues that historians might use instruments they’re not educated to grasp. “I believe there’s nice threat in it, as a result of we as humanists or historians are successfully outsourcing evaluation to a different subject, or maybe a machine,” says Abraham Gibson, a historical past professor on the College of Texas at San Antonio. Gibson says till very lately, fellow historians he spoke to didn’t see the relevance of synthetic intelligence to their work, however they’re more and more waking as much as the chance that they may ultimately yield among the interpretation of historical past to a black field.
This “black field” drawback shouldn’t be distinctive to historical past: even builders of machine-learning methods generally wrestle to grasp how they perform. Happily, some strategies designed with historians in thoughts are structured to offer better transparency. Ithaca produces a spread of hypotheses ranked by chance, and BIFOLD researchers are engaged on the interpretation of their fashions with explainable AI, which is supposed to disclose which inputs contribute most to predictions. Historians say they themselves promote transparency by encouraging individuals to view machine studying with vital detachment: as a great tool, however one which’s fallible, similar to individuals.
The historians of tomorrow
Whereas skepticism towards such new expertise persists, the sphere is progressively embracing it, and Valleriani thinks that in time, the variety of historians who reject computational strategies will dwindle. Students’ issues in regards to the ethics of AI are much less a cause to not use machine studying, he says, than a possibility for the humanities to contribute to its growth.
Because the French historian Emmanuel Le Roy Ladurie wrote in 1968, in response to the work of historians who had began experimenting with computational historical past to analyze questions akin to voting patterns of the British parliament within the 1840s, “the historian of tomorrow will likely be a programmer, or he is not going to exist.”
Moira Donovan is an unbiased science journalist primarily based in Halifax, Nova Scotia.