On a desk in his lab on the College of Pennsylvania, physicist Sam Dillavou has linked an array of breadboards through an online of brightly coloured wires. The setup seems like a DIY residence electronics venture—and never a very elegant one. However this unassuming meeting, which comprises 32 variable resistors, can study to kind knowledge like a machine-learning mannequin.
Whereas its present functionality is rudimentary, the hope is that the prototype will supply a low-power various to the energy-guzzling graphical processing unit (GPU) chips broadly utilized in machine studying.
“Every resistor is easy and sort of meaningless by itself,” says Dillavou. “However whenever you put them in a community, you possibly can prepare them to do a wide range of issues.”
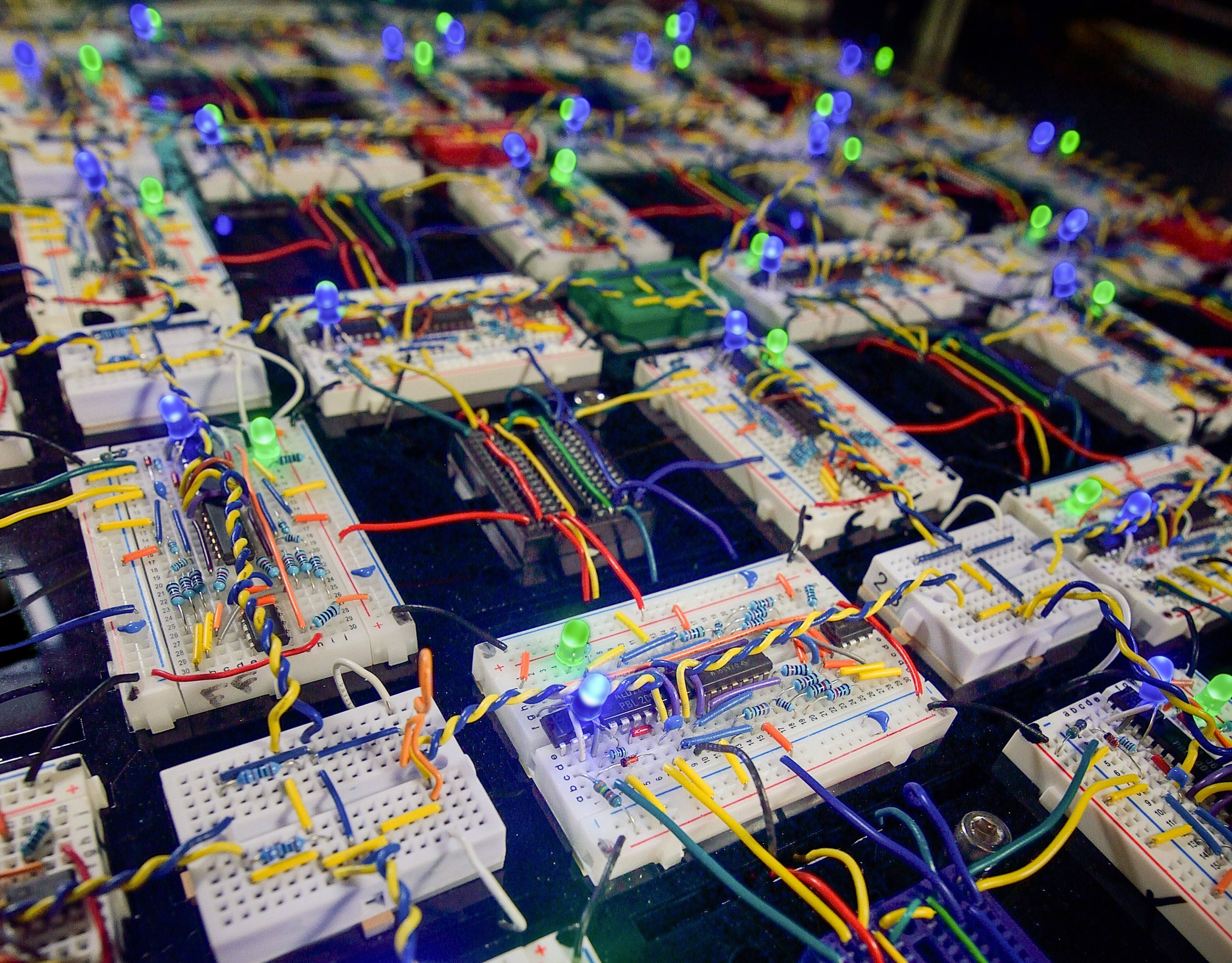
A activity the circuit has carried out: classifying flowers by properties similar to petal size and width. When given these flower measurements, the circuit might kind them into three species of iris. This type of exercise is named a “linear” classification drawback, as a result of when the iris info is plotted on a graph, the information will be cleanly divided into the right classes utilizing straight traces. In follow, the researchers represented the flower measurements as voltages, which they fed as enter into the circuit. The circuit then produced an output voltage, which corresponded to one of many three species.
This can be a basically totally different method of encoding knowledge from the strategy utilized in GPUs, which characterize info as binary 1s and 0s. On this circuit, info can tackle a most or minimal voltage or something in between. The circuit categorised 120 irises with 95% accuracy.
Now the staff has managed to make the circuit carry out a extra complicated drawback. In a preprint at present beneath evaluation, the researchers have proven that it might carry out a logic operation often known as XOR, during which the circuit takes in two binary numbers and determines whether or not the inputs are the identical. This can be a “nonlinear” classification activity, says Dillavou, and “nonlinearities are the key sauce behind all machine studying.”
Their demonstrations are a stroll within the park for the gadgets you utilize every single day. However that’s not the purpose: Dillavou and his colleagues constructed this circuit as an exploratory effort to search out higher computing designs. The computing business faces an existential problem because it strives to ship ever extra highly effective machines. Between 2012 and 2018, the computing energy required for cutting-edge AI fashions elevated 300,000-fold. Now, coaching a big language mannequin takes the identical quantity of power because the annual consumption of greater than 100 US properties. Dillavou hopes that his design presents an alternate, extra energy-efficient strategy to constructing sooner AI.
Coaching in pairs
To carry out its varied duties accurately, the circuitry requires coaching, identical to modern machine-learning fashions that run on typical computing chips. ChatGPT, for instance, discovered to generate human-sounding textual content after being proven many cases of actual human textual content; the circuit discovered to foretell which measurements corresponded to which sort of iris after being proven flower measurements labeled with their species.
Coaching the gadget entails utilizing a second, equivalent circuit to “instruct” the primary gadget. Each circuits begin with the identical resistance values for every of their 32 variable resistors. Dillavou feeds each circuits the identical inputs—a voltage comparable to, say, petal width—and adjusts the output voltage of the second circuit to correspond to the right species. The primary circuit receives suggestions from that second circuit, and each circuits regulate their resistances in order that they converge on the identical values. The cycle begins once more with a brand new enter, till the circuits have settled on a set of resistance ranges that produce the right output for the coaching examples. In essence, the staff trains the gadget through a way often known as supervised studying, the place an AI mannequin learns from labeled knowledge to foretell the labels for brand spanking new examples.
It could possibly assist, Dillavou says, to think about the electrical present within the circuit as water flowing via a community of pipes. The equations governing fluid circulate are analogous to these governing electron circulate and voltage. Voltage corresponds to fluid strain, whereas electrical resistance corresponds to the pipe diameter. Throughout coaching, the totally different “pipes” within the community regulate their diameter in varied components of the community to be able to obtain the specified output strain. The truth is, early on, the staff thought-about constructing the circuit out of water pipes relatively than electronics.
For Dillavou, one fascinating side of the circuit is what he calls its “emergent studying.” In a human, “each neuron is doing its personal factor,” he says. “After which as an emergent phenomenon, you study. You might have behaviors. You experience a motorbike.” It’s comparable within the circuit. Every resistor adjusts itself based on a easy rule, however collectively they “discover” the reply to a extra sophisticated query with none specific directions.
A possible power benefit
Dillavou’s prototype qualifies as a sort of analog laptop—one which encodes info alongside a continuum of values as a substitute of the discrete 1s and 0s utilized in digital circuitry. The primary computer systems have been analog, however their digital counterparts outmoded them after engineers developed fabrication strategies to squeeze extra transistors onto digital chips to spice up their pace. Nonetheless, consultants have lengthy identified that as they enhance in computational energy, analog computer systems supply higher power effectivity than digital computer systems, says Aatmesh Shrivastava, {an electrical} engineer at Northeastern College. “The ability effectivity advantages should not up for debate,” he says. Nevertheless, he provides, analog alerts are a lot noisier than digital ones, which make them in poor health fitted to any computing duties that require excessive precision.
In follow, Dillavou’s circuit hasn’t but surpassed digital chips in power effectivity. His staff estimates that their design makes use of about 5 to 20 picojoules per resistor to generate a single output, the place every resistor represents a single parameter in a neural community. Dillavou says that is a few tenth as environment friendly as state-of-the-art AI chips. However he says that the promise of the analog strategy lies in scaling the circuit up, to extend its variety of resistors and thus its computing energy.
He explains the potential power financial savings this manner: Digital chips like GPUs expend power per operation, so making a chip that may carry out extra operations per second simply means a chip that makes use of extra power per second. In distinction, the power utilization of his analog laptop relies on how lengthy it’s on. Ought to they make their laptop twice as quick, it might additionally change into twice as power environment friendly.
Dillavou’s circuit can be a sort of neuromorphic laptop, which means one impressed by the mind. Like different neuromorphic schemes, the researchers’ circuitry doesn’t function based on top-down instruction the way in which a standard laptop does. As a substitute, the resistors regulate their values in response to exterior suggestions in a bottom-up strategy, just like how neurons reply to stimuli. As well as, the gadget doesn’t have a devoted element for reminiscence. This might supply one other power effectivity benefit, since a standard laptop expends a major quantity of power shuttling knowledge between processor and reminiscence.
Whereas researchers have already constructed a wide range of neuromorphic machines based mostly on totally different supplies and designs, probably the most technologically mature designs are constructed on semiconducting chips. One instance is Intel’s neuromorphic laptop Loihi 2, to which the corporate started offering entry for presidency, tutorial, and business researchers in 2021. DeepSouth, a chip-based neuromorphic machine at Western Sydney College that’s designed to have the ability to simulate the synapses of the human mind at scale, is scheduled to return on-line this 12 months.
The machine-learning business has proven curiosity in chip-based neuromorphic computing as nicely, with a San Francisco–based mostly startup referred to as Rain Neuromorphics elevating $25 million in February. Nevertheless, researchers nonetheless haven’t discovered a industrial software the place neuromorphic computing definitively demonstrates a bonus over typical computer systems. Within the meantime, researchers like Dillavou’s staff are placing forth new schemes to push the sphere ahead. A couple of folks in business have expressed curiosity in his circuit. “Individuals are most within the power effectivity angle,” says Dillavou.
However their design continues to be a prototype, with its power financial savings unconfirmed. For his or her demonstrations, the staff stored the circuit on breadboards as a result of it’s “the best to work with and the quickest to alter issues,” says Dillavou, however the format suffers from all types of inefficiencies. They’re testing their gadget on printed circuit boards to enhance its power effectivity, and so they plan to scale up the design so it might carry out extra sophisticated duties. It stays to be seen whether or not their intelligent concept can take maintain out of the lab.