We’re witnessing a historic, world paradigm shift pushed by dramatic enhancements in AI. As AI has advanced from predictive to generative, extra companies are taking discover, with enterprise adoption of AI greater than doubling since 2017. In response to McKinsey, 63% of respondents anticipate their organizations’ funding in AI to extend over the subsequent three years.
Paralleling this unprecedented adoption of AI, the amount of compute can be rising at a shocking price. Since 2012, the quantity of compute used within the largest AI coaching runs has grown by greater than 300,000 occasions. But, as sizable computing calls for develop, important environmental implications observe.
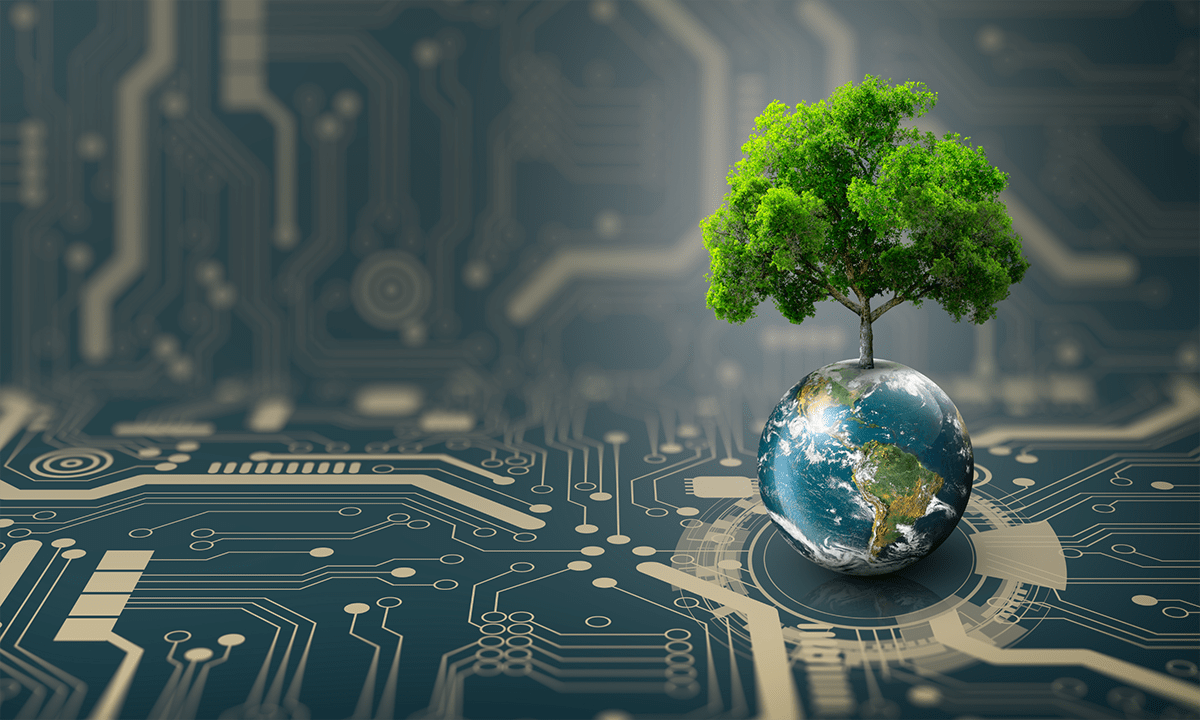
Extra compute results in larger electrical energy consumption, and consequent carbon emissions. A 2019 examine by researchers on the College of Massachusetts Amherst estimated that the electrical energy consumed throughout the coaching of a transformer, a sort of deep studying algorithm, can emit greater than 626,000 kilos (~284 metric tons) of carbon dioxide—equal to greater than 41 round-trip flights between New York Metropolis and Sydney, Australia. And that’s simply coaching the mannequin.
We’re additionally going through an explosion of information storage. IDC tasks that 180 zettabytes of information—or, 180 billion terabytes—might be created in 2025. The collective power required for knowledge storage at this scale is big and might be difficult to deal with sustainably. Relying on the circumstances of information storage (e.g., {hardware} used, power mixture of the ability), a single terabyte of saved knowledge can produce 2 tons of CO2 emissions yearly. Now multiply that by 180 billion.
This present trajectory for intensifying AI with an ever-growing environmental footprint is solely not sustainable. We have to rethink the established order and alter our methods and habits.
Driving sustainable enhancements with AI
Whereas there are undoubtedly critical carbon emissions implications with the elevated prominence of AI, there are additionally monumental alternatives. Actual-time knowledge assortment mixed with AI can truly assist companies shortly determine areas for operational enchancment to assist scale back carbon emissions at a scale.
For instance, AI fashions can determine instant enchancment alternatives for elements influencing constructing effectivity, together with heating, air flow, and air-con (HVAC). As a posh, data-rich, multi-variable system, HVAC is well-suited to automated optimization, and enhancements can result in power financial savings inside just some months. Whereas this chance exists in nearly any constructing, it’s particularly helpful in knowledge facilities. A number of years in the past, Google shared how implementing AI to enhance knowledge heart cooling diminished its power consumption by as much as 40%.
AI can be proving efficient for implementing carbon-aware computing. Routinely shifting computing duties, based mostly on the provision of renewable power sources, can decrease the carbon footprint of the exercise.
Likewise, AI may also help diminish the ballooning knowledge storage downside beforehand talked about. To handle the sustainability issues of large-scale knowledge storage, Gerry McGovern, in his e-book World Huge Waste, acknowledged that as much as 90% of information is unused—merely saved. AI may also help decide what knowledge is effective, crucial, and of excessive sufficient high quality to warrant storage. Superfluous knowledge can merely be discarded, saving each value and power.
Find out how to design AI tasks extra sustainably
To responsibly implement AI initiatives, all of us have to rethink just a few issues and take a extra proactive method to designing AI tasks.
Start with a crucial examination of the enterprise downside you are attempting to resolve. Ask: Do I actually need AI to resolve this downside or can conventional probabilistic strategies with decrease computing and power necessities suffice? Deep studying just isn’t the answer to all issues, so it pays to be selective when making the willpower.
When you’ve clarified your online business downside or use case, fastidiously think about the next when developing your answer and mannequin:
- Emphasize knowledge high quality over knowledge amount. Smaller datasets require much less power for coaching and have lighter ongoing compute and storage implications, thereby producing fewer carbon emissions. Research present that most of the parameters inside a skilled neural community will be pruned by as a lot as 99%, yielding a lot smaller, extra sparse networks.
- Think about the extent of accuracy actually wanted to resolve in your use case. As an example, if you happen to had been to fine-tune your fashions for a decrease accuracy consumption calculation, quite than compute-intensive FP32 calculations, you may drive important power financial savings.
- Leverage domain-specific fashions and cease re-inventing the wheel. Orchestrating an ensemble of fashions from present, skilled datasets can provide you higher outcomes. For instance, if you have already got a big mannequin skilled to grasp language semantics, you may construct a smaller, domain-specific mannequin tailor-made to your wants that faucets into the bigger mannequin’s information base, leading to related outputs with far more effectivity.
- Steadiness your {hardware} and software program from edge to cloud. A extra heterogenous AI infrastructure, with a mix of AI computing chipsets that meet particular utility wants, will make sure you save power throughout the board, from storage to networking to compute. Whereas edge gadget SWaP (dimension, weight, and energy) constraints require smaller, extra environment friendly AI fashions, AI calculations nearer to the place knowledge is generated may end up in extra carbon-efficient computing with lower-power units and smaller community and knowledge storage necessities. And, for devoted AI {hardware}, utilizing built-in accelerator applied sciences to extend efficiency per watt can yield important power financial savings. Our testing exhibits built-in accelerators can enhance common efficiency per watt effectivity 3.9x on focused workloads when in comparison with the identical workloads operating on the identical platform with out accelerators. (Outcomes might fluctuate.)
- Think about open-source options with libraries of optimizations to assist make sure you’re getting the most effective efficiency out of your {hardware} and frameworks out of the field. Along with open supply, embracing open requirements may also help with repeatability and scale. For instance, to keep away from energy-intensive preliminary mannequin coaching, think about using pre-trained fashions for larger effectivity and the potential for shared/federated learnings and enhancements over time. Equally, open APIs allow extra environment friendly cross-architecture options, permitting you to construct instruments, frameworks, and fashions as soon as and deploy in all places with extra optimum efficiency.
Like many sustainability-led choices, designing your AI tasks to scale back their environmental influence just isn’t straightforward. Lowering your power and carbon footprint requires work, intention, and compromise to take advantage of accountable selections. However as we see in different sustainability-led enterprise selections, even seemingly small changes can create giant, collective enhancements to scale back carbon emissions and assist gradual the consequences of local weather change.
To study extra about how Intel may also help you attain your sustainable computing objectives, go to Intel.com/sustainability.
This content material was produced by Intel. It was not written by MIT Know-how Overview’s editorial workers.